Back to School with AI, Part 4: AI and the Question of Rigor
If students need to struggle in order to learn, why would we encourage them to use AI?
Earlier this week, I had the pleasure of presenting on generative artificial intelligence to two groups in Atlanta, GA, USA: the faculty of Woodward Academy and members of the Atlanta Area Technology Educators (AATE) network. My favorite part of these kinds of events is hearing the questions people ask. Questions are the best data: they reveal not just what people want to know, but what they are worrying about, what they are excited about, and how they are thinking about the topic at hand.
There’s a section in one of my workshops that begins with the question, “How might students use AI to help them think?” I offer some examples of ways students could use AI for learning, not instead of learning. Soon after I finished this section, a member of the audience asked a question (I’m paraphrasing it):
If students need to struggle in order to learn, why would we encourage them to use AI?
I love this question. It gets to the heart of what causes learning. I’ve been thinking about it since that session and wanted to expand on the answer I gave at the time (which I hope was helpful!).
We often conflate struggle with rigor: that in order for students to learn, they need to do things that are hard. The problem with the term rigor is that it is too often associated with output (pages read, hours studied, courses taken, levels completed). At its worst, rigor is difficulty without purpose, more about assessing what students can endure rather than what they have learned.
Yet research on learning suggests inputs (meaningful tasks that are aligned to students’ prior knowledge and responsive to student values, culture, and identity) and process (scaffolding, differentiation, feedback) are more important when it comes to ensuring students feel challenged and supported so that they can learn. Outputs are simply how we assess how well students used the inputs to navigate the learning process.
If we look at Lev Vygotsky's Zone of Proximal Development (ZPD) or Mihaly Csikszentmihalyi’s concept of Flow, students learn when they take on challenging tasks that are emotionally satisfying and involve the guidance of a skilled partner like a teacher, tutor, or peer.
I like this simple rendering of the concepts:
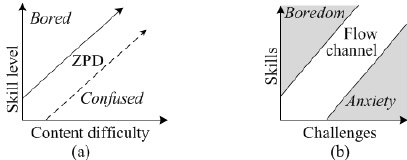
Designing learning experiences for challenge is different than simply making things hard. There are both cognitive and noncognitive components to what enables students to succeed at challenging tasks before veering into confusion or anxiety. Cognitive load theory explains that the brain has limited capacity to process new information, and it will stop processing it once overwhelmed. Camille Farrington’s research (among many other examples) shows belonging, relevance, and confidence in success all contribute to deeper learning because they are factors in 1) the student’s trust in the teacher and in the value of the task and 2) their motivation to try something new or hard. The complexity of teaching is guiding students to the right places in the learning process where they can focus their precious cognitive and emotional energy on the tasks that will increase knowledge and competence. Aiming for struggle through rigor without considering emotional satisfaction and meaningful support undermines the process.
AI and Bloom’s Taxonomy
The question that interests me when it comes to AI and learning is: can AI improve a student’s chances of finding the right zone for learning? Let’s start with Bloom’s Taxonomy:
1. AI can be a guide. AI can already perform many of the functions that a tutor or a skilled peer or a parent can when it comes to providing the guidance students need to find the right zone for learning.
Remember: AI can assist a student in memorizing vocabulary or formulas by learning and presenting the information in a variety of formats.
Understand: AI can assist a student with processing information by organizing or explaining it in different ways.
Apply: AI can model a potential way to solve a problem in a way that allows the student to create their own solution and compare it to AI’s.
Analyze: AI can assist students with analysis by reviewing their work and asking follow-up questions.
Evaluate: AI can assist students by generating alternative arguments or perspectives for the student to critique.
Create: AI can assist students in creation by offering feedback and elaboration on their new ideas.
We are already seeing studies that suggest that using AI for complex knowledge work improves performance over not using it and is particularly effective at accelerating improvement for lower-level performers. Perhaps using AI knocks down some of the cognitive and emotional barriers that hold these performers back from learning deeply. Perhaps it offers assistance not previously accessible to them (like human tutors). Perhaps it smooths out the simpler or less important parts of the process to ensure they’re fresh for complex tasks. The cause might not be clear, but the impact is already apparent: AI can help us work at all levels of Bloom’s.
2. Learning to use AI is itself a productive struggle. In his excellent newsletter “Intentional Teaching” (you can subscribe here), Derek Bruff writes, “Something I've observed about the use of generative AI for hard tasks, at least so far, is that experts can coach an AI to produce expert-level work, but novices in a particular domain cannot…As teachers our job is to help our students develop expertise whether or not those students use AI technologies along the way. The really interesting question for me is, where can generative AI help develop that expertise?”
I find it so much more compelling to consider how to design for expertise than for rigor. Learning how to use AI is challenging (in the good way) because of the critical thinking involved in analyzing responses, creating prompts, and understanding how to train AI through feedback and dialogue. Using it well also involves avoiding the negative effects of regular use (see the same study I cited above) that can stifle critical thinking. Negotiating these differences requires guided practice.
If using AI well involves understanding how experts in a field think and act, why wouldn’t we collaborate with students to use AI to develop their understanding of what expertise requires?
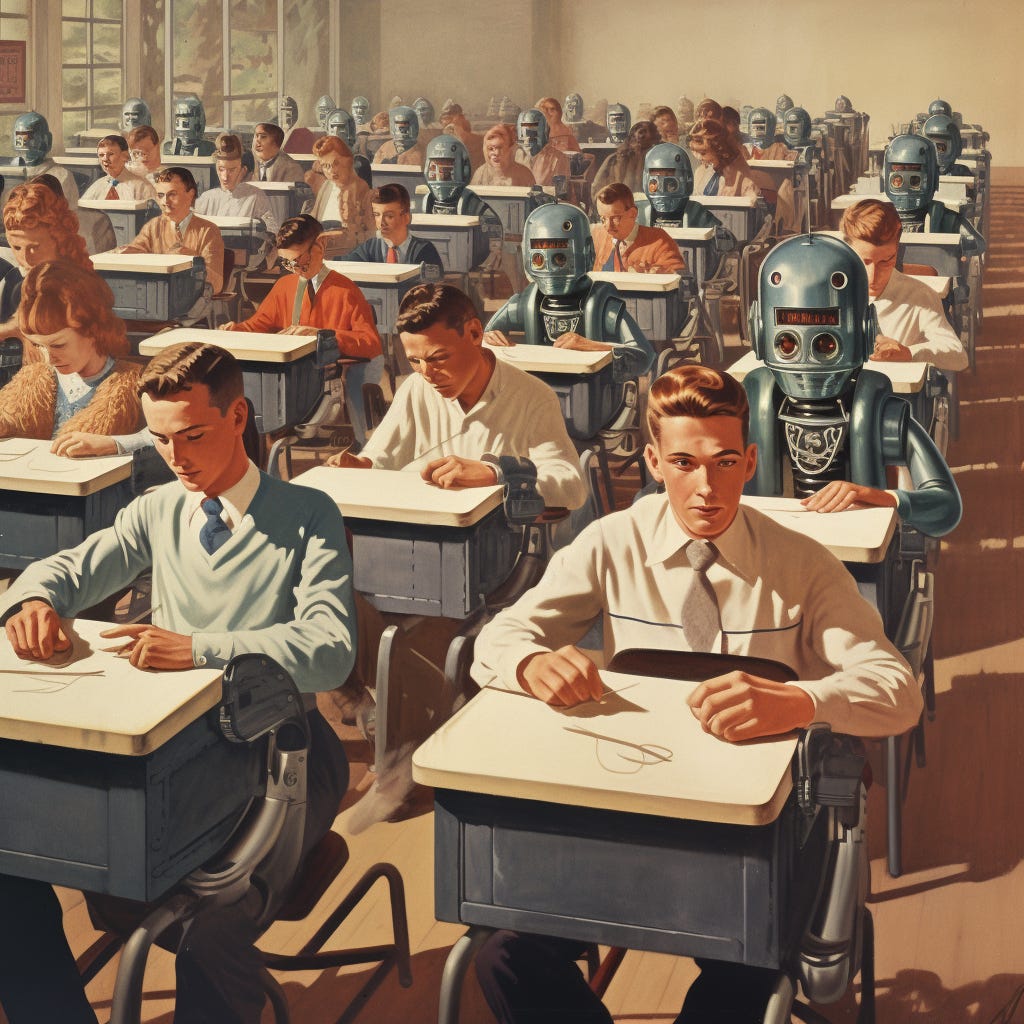
Augmentation, not Automation. Assistance, not Cheating.
In his 2022 paper “The Turing Trap,” Erik Brynjolfsson of Stanford University argues that the real potential of AI lies not in its ability to automate human tasks (thus, replacing the need for humans), but rather in its ability to augment human’s abilities, to help us be better at what we can do now and to enable us to extend our abilities in ways we haven’t conceived of yet.
This framing reminded me of what I heard teacher Cherie Shields say on the Hard Fork podcast back in January, including a line that has become a mantra for me when I talk about AI.
Like so many technological innovations before it—I would argue going all the way back to the printing press up to more recent phenomena like calculators and the internet and Google Translate—AI is shifting the boundaries of what we might think of as “cheating” (automating or replacing learning) and what we might think of as “assistance” (augmenting learning). But, the boundaries are unclear and evolving, and finding those boundaries begins with a conversation that helps us develop a shared understanding of what “cheating” and “assistance” mean to us right now and in a future that is going to involve more sophisticated AI.
Sarah Hanawald of the Association for Academic Leaders shared this terrific activity from Ditch That Textbook in her AATE workshop in Atlanta. These prompts would make a wonderful agenda for a department or grade level meeting or, more importantly to me, a conversation with students:
These are hard—one might even say rigorous—conversations that could (and probably will) lead to us to audit our current assessments: eliminating some, revising others, and creating new ones. But, I hope these conversations focus on what we know students need in order to learn, and how AI can help them and us find a process that is meaningful and supportive.
What about us?
As a final thought, here are some examples of how educators in Atlanta told me they were using AI to support their work:
A teacher used a chatbot to review a previous assessment of hers, identify and explain the essential vocabulary students would need to learn to do well in that assessment, then generate new assessments and activities for students to learn that vocabulary.
A teacher downloaded transcripts of instructional videos he uses in class, then uploaded them into a chatbot and asked it to generate guided notes for students.
A teacher used a chatbot to increase the number of quiz questions he can make available to students.
A teacher used a chatbot to generate new ideas for curriculum and lessons.
A teacher created an app built off of ChatGPT that asked a series of questions of a teacher and then used the responses to compose a college recommendation letter.
Are any of these teachers “cheating”? Does their use of AI diminish the rigor of their work? Why or why not? Should we hold students to the same standard? Or a different one?
I’d love to hear what you think.
Upcoming Workshops
Join me for some live learning about AI:
I’m facilitating an online workshop called “Making Sense of AI” with the California Teacher Development Collaborative on Tuesday, September 26, at 3:30pm Pacific Time.Registration is open until Monday. You can sign up even if you don’t live/work in California!I’ll be facilitating a workshop on AI and assessment at theInnovative Learning Conferenceat the Nueva School in San Mateo, CA, USA, October 26-27.
Links!
The American School in Japan has released its “Guidelines and Guardrails, Permissions and Constraints” for use of AI at the school. I really like their approach and the result. It’s worth reading this article from the student newspaper before diving into the document itself.
Educators in Atlanta were talking up a couple of AI tools: MagicSchool for helping teacher workflow and ClipDrop for image editing.
Students at The Downtown School in Seattle, WA, USA, got to meet with researchers at the University of Washington who are designing ways AI can help humans make ethical decisions. Teacher George Heinrichs used their Value Kaleidoscope to think through grading practices.
More links! Anna Mills curated this excellent reading list of pieces by researchers on the potentials and pitfalls of AI.
Thanks for reading! If you enjoyed this post, I hope you’ll share it with others. It’s free to subscribe to Learning on Purpose. If you have feedback or just want to connect, you can always reach me at eric@erichudson.co.
Hi, Eric,
Impressive content. Love the visuals! A very persuasive case.
We are working on similar issues. I'd appreciate it if you had a moment to check out my substack:
https://nickpotkalitsky.substack.com?utm_source=navbar&utm_medium=web&r=2l25hp
Be well. Glad I found your newsletter. Can't wait to read more.
Nick
It was an attendee at the event who created Letterwise, a generative ai tool for writing college recommendation letters. It's existence and the use of it has helped me kick off some fascinating conversations. https://letterwise.us/